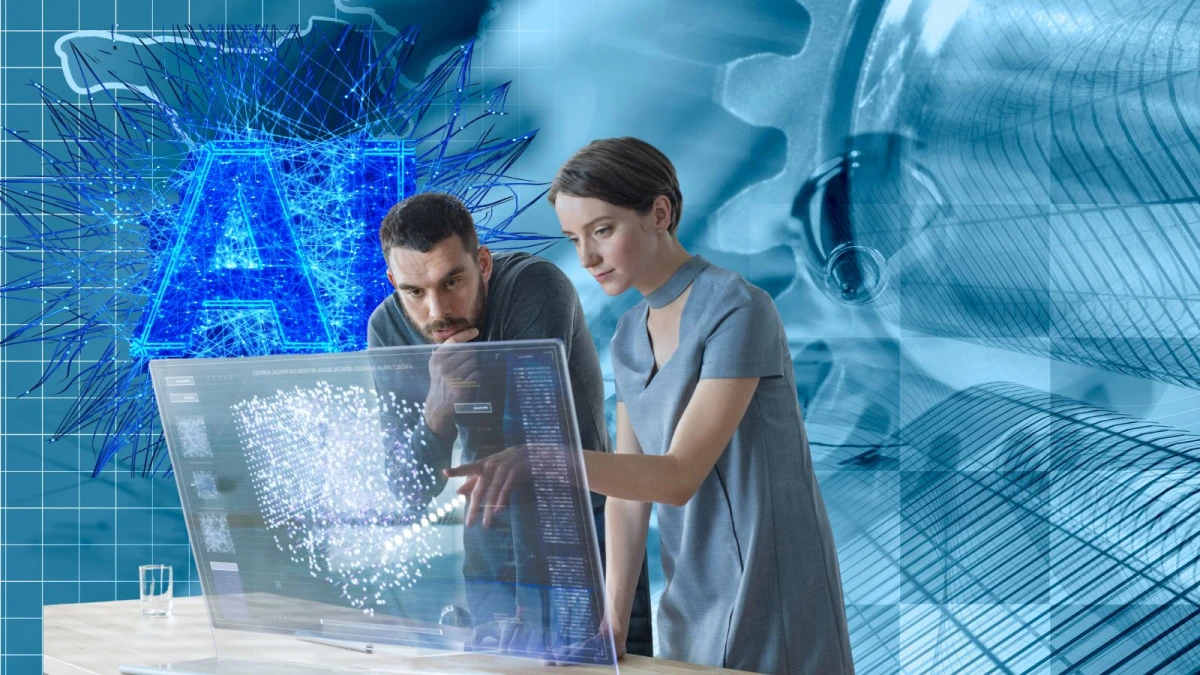
Practical Guide for LLMs in the Financial Industry Introduction
Large language models (LLMs) are advanced artificial intelligence (AI) models trained to understand and generate human-like text based on vast datasets, often containing millions or even billions of sentences. At the core of LLMs are deep neural networks that learn patterns, relationships, and contextual nuances in language. By processing sequences of words, phrases, and sentences, these models can predict and generate coherent responses, answer questions, create summaries, and even carry out complex, specialized tasks.
In the financial industry, the adoption of LLMs is still in its early stages, but interest is rapidly growing. Financial institutions are beginning to explore how these models can enhance various processes, such as analyzing financial reports, automating customer service, detecting fraud, and conducting market sentiment analysis. While some organizations are experimenting with these technologies, widespread integration is limited due to such factors as data privacy concerns, regulatory compliance, and the need for specialized fine-tuning to ensure accuracy in finance-specific applications.
In response to these challenges, many organizations are adopting a hybrid approach that combines frontier large-scale LLMs with retrieval-augmented generation (RAG) systems.1 This approach leverages the strengths of LLMs for general language understanding while incorporating domain-specific data through retrieval mechanisms to improve accuracy and relevance. However, the value of smaller, domain-specific models remains significant, especially for tasks requiring efficient processing or where data privacy and regulatory compliance are of utmost concern. These models offer tailored solutions that can be fine-tuned to meet the stringent demands of the financial industry, providing a complementary alternative to larger, more generalized systems.
[....]